Title: Biomanufacturing Digital Twin Modeling and Machine Learning Ontology
Highlights of a NIST ontology project (ongoing):
-
Problem Statement. Biopharmaceutical manufacturing faces critical challenges, including high complexity,
limited data, and fast evolution of biotech (e.g., new bio-drugs and sensor technologies frequently coming to the market).
-
Technology Development. To address the challenges and facilitate Industry 4.0, the objective of this project is to develop
a mechanistic modeling and machine learning (ML) ontology platform that can enhance interoperability, reuse mechanistic models and
data from various sources, and support flexible, optimal, automated and regulated biomanufacturing.
1) Develop an ontologically encoded multi-scale foundation model and create a knowledge graph (KG) with a modular and hierarchical design that can decode digital twins and mechanistic models, integrate experimental data from different sources, and support interoperability of cell culture manufacturing processes.
2) Construct a ML ontology on the KG focusing on sensitivity analysis (SA) and gradient optimization algorithms that will model the digital thread of model development, training, prediction, and optimal control. This enables users to systematically learn mechanistic parameters and process control strategies.
3) Validate the proposed ¡°KG + ML¡± ontology platform with advanced query and reasoning function to automatically support biomanufacturing systems quality control and root-cause analysis.
- Projected Impacts.
This modeling and ML platform will pave the way to establishing a unified ontology and general digital twin framework
supporting the evolving needs of biomanufacturing domain and enhancing data interoperability and analysis.
NIIMBL 4.1W: Modularized PAT Online Training Platform to Accelerate the Workforce Innovation in Biopharmaceuticals Manufacturing
The video demonstrates interactive interfaces (still under development). It includes
1) A plant-wide simulator generating experimental trajectories of integrated bioreactor cell culture and chromatography purification processes.
2) A Raman spectroscopy simulator taking state concentrations from the plant-wide simulator as inputs to produce Raman spectra.
3) Risk-based ML/PAT training modules including root cause analysis, DoE, integrated bioprocess monitoring, prediction, and robust control.
Highlights of project NIIMBL 4.1W
-
Problem Statement. In the project, we create a modularized extensible online training platform on
leading-edge process analytical
technologies (PAT).
It can provide large-scale, low-cost, and high-quality life-long customized training, support workforce innovation, facilitate biomanufacturing 4.0, and strengthen the competitive advantage and leading position of the U.S. in innovative biopharmaceutical development and manufacturing. -
Technology Development. Integrating with professional training certification programs on process control, design
of experiments, and data analytics, we create new PAT training materials and tools including:
1) end-to-end biopharmaceutical manufacturing process hybrid models, which can leverage the existing mechanistic models from each unit operation, provide science- and risk-based production process understanding, and quantify the spatiotemporal causal interdependencies of critical process parameters (CPPs) and critical quality attributes (CQAs);
2) bioprocess sensitivity and risk analyses, which can guide process specifications and troubleshooting, reduce release times, and support quality-by-design (QbD);
3) risk-based prediction, which can provide reliable guidance on dynamic decision-making, accelerate process development, and support integrated production process automation. In addition, we develop the digital twin-based virtual lab (vLab) and real problem-derived case studies to reinforce mechanistic knowledge, support understanding of bioprocess uncertainties, provide experiential learning, and facilitate problem-solving skills development. - Projected Impacts.
1) The proposed PAT online training platform can enrich the current education programs (i.e., biotechnology, biomanufacturing, machine learning) with risk-based integrated bioprocess modeling/analysis/prediction, interpretable Artificial intelligence (AI), and digital twins, which can facilitate end-to-end biomanufacturing self-learning and automation.
2) The platform can instruct trainees with diverse backgrounds (i.e., college undergraduate and graduate students, current industry practitioners and government regulators, associate degree students in community colleges) to support their career development in current and future biopharmaceuticals manufacturing.
3) The platform can support the biopharmaceutical manufacturing innovations by: (i) minimizing human errors and defects; (ii) accelerating QbD, process specification, and real-time release; and (iii) facilitating the move to modular, efficient, robust, flexible, reliable, intensified, and automated biomanufacturing.
Optical Redox Probe for Continuous Metabolic Monitoring during Natural Products Bioprocessing
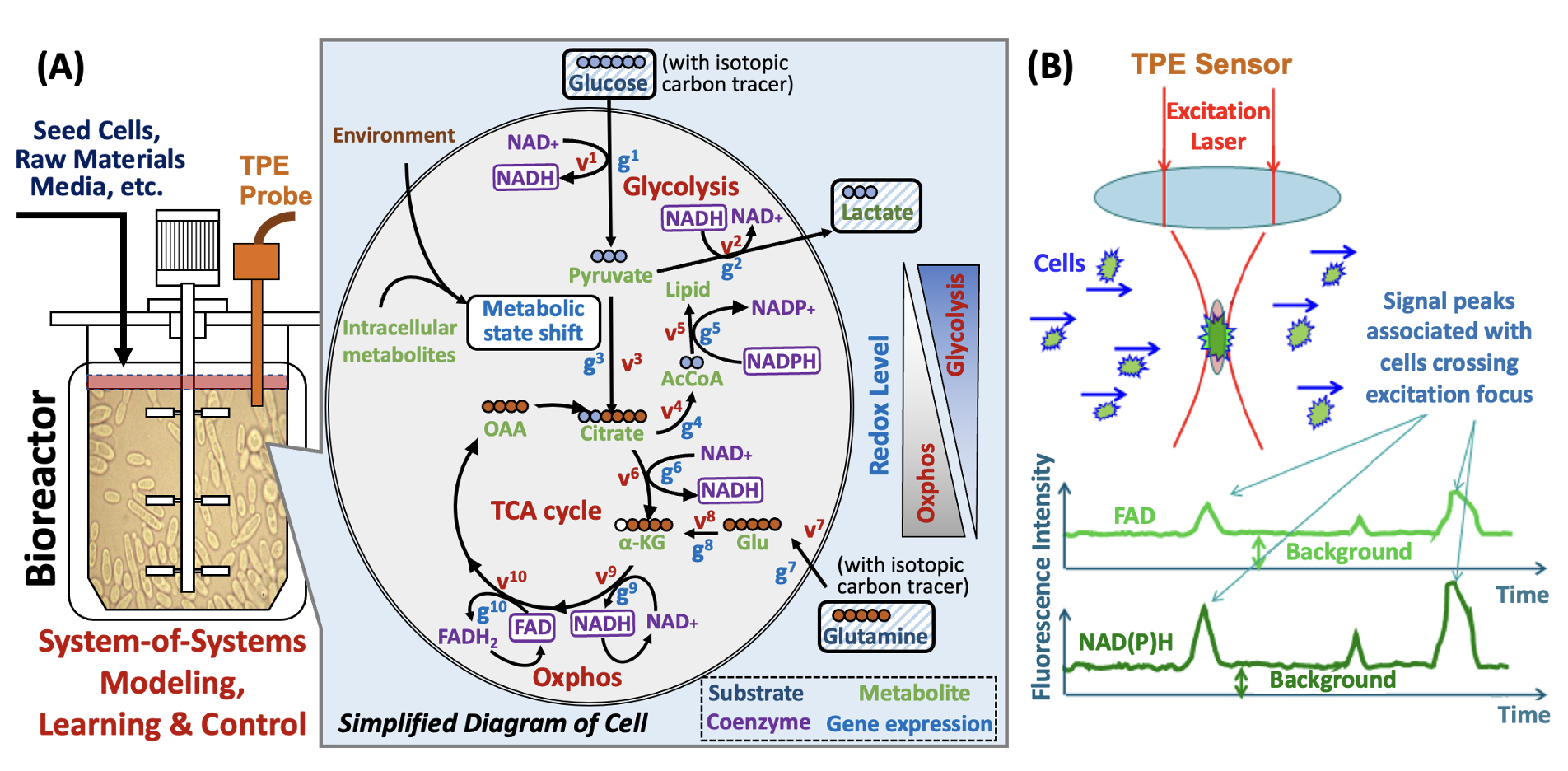
(A) The proposed multi-scale cell culture mechanistic model and soft sensor can integrate heterogenous multi-omics and TPE probe measures to enable the inference of metabolic state of yeast cells, identify intrinsic apoptosis pathways activated in response to hypoxia and nutrient starvation, and guide the selection of bioprocess optimal control strategy to improve productivity and facilitate continuous biomanufacturing. (B) The TPE sensor can provide real-time measurements on intracellular metabolite NAD(P)H/FAD and redox levels that can be used to monitor metabolic state change from glycolysis to oxidative phosphorylation (Oxphos). Since cells are bigger than the focal volume, when a cell flows through the focus, the background emission from other fluorescent components is negligible.
Highlights of a NIH project on Optical Probe and Metabolic Monitoring (ongoing):
-
Problem Statement.
The objective of this project is to develop and validate a novel optical sensing technology that enables on-line, continuous measurement of cellular-level metabolism of reacting microorganisms and cells in bioreactors.
-
Technology Development.
The proposed two-photon excitation (TPE) fluorescence probe for real-time measurement of important metabolites
within individual cells will guide the development of efficient biomanufacturing of natural products (e.g.,
medicines, biofuels, and foods). We are creating cell culture mechanistic model and process analytical technology
(PAT) to evaluate and demonstrate the merit of the new metabolic data in guiding and promoting the development of
effective bioreactor feeding and control strategies (e.g., glucose feeding and oxygen control) for improved biosynthesis.
1) Create an interpretable probabilistic knowledge graph (KG) hybrid model characterizing the science- and risk-based understanding of cell culture and fermentation process mechanisms at molecular, cellular, and system levels.
2) This model will: a) relate the real-time redox states to the key bioreactor operation parameters (e.g., glucose level, oxygen level); and b) fully utilize the cellular-level real-time redox data from TPE probe to improve the prediction of metabolic state change and fermentation productivity.
3) Evaluate the value of real-time individual cell metabolism monitoring data to improve the understanding of biosynthesis processes and improve bioreactor operations.
4) Develop a knowledge graph assisted reinforcement learning (RL) framework to guide the optimal sequential design of experiments to accelerate the process development/control and improve the productivity.
5) The developed real-time redox monitoring and metabolic state informed control strategy will be tested during adjusted fed-batch and continuous bioreactor operations and further extended to a long-term continuous biomanufacturing process (with operation for four weeks or longer). - Projected Impacts. The accurate intracellular metabolism measurement data and the validated PATs provided by this project will be valuable for bioreactor operators to select effective feeding strategies, for upstream scientists to develop advanced process technologies, and for microbiologists to discover new metabolism-engineered species and re-engineer the biosynthesis pathways.
NIIMBL 5.2T: Advanced Bioprocess Sensor and Analytical Technologies for Induced Pluripotent Stem Cell (iPSC) Culture Online Monitoring and Automation
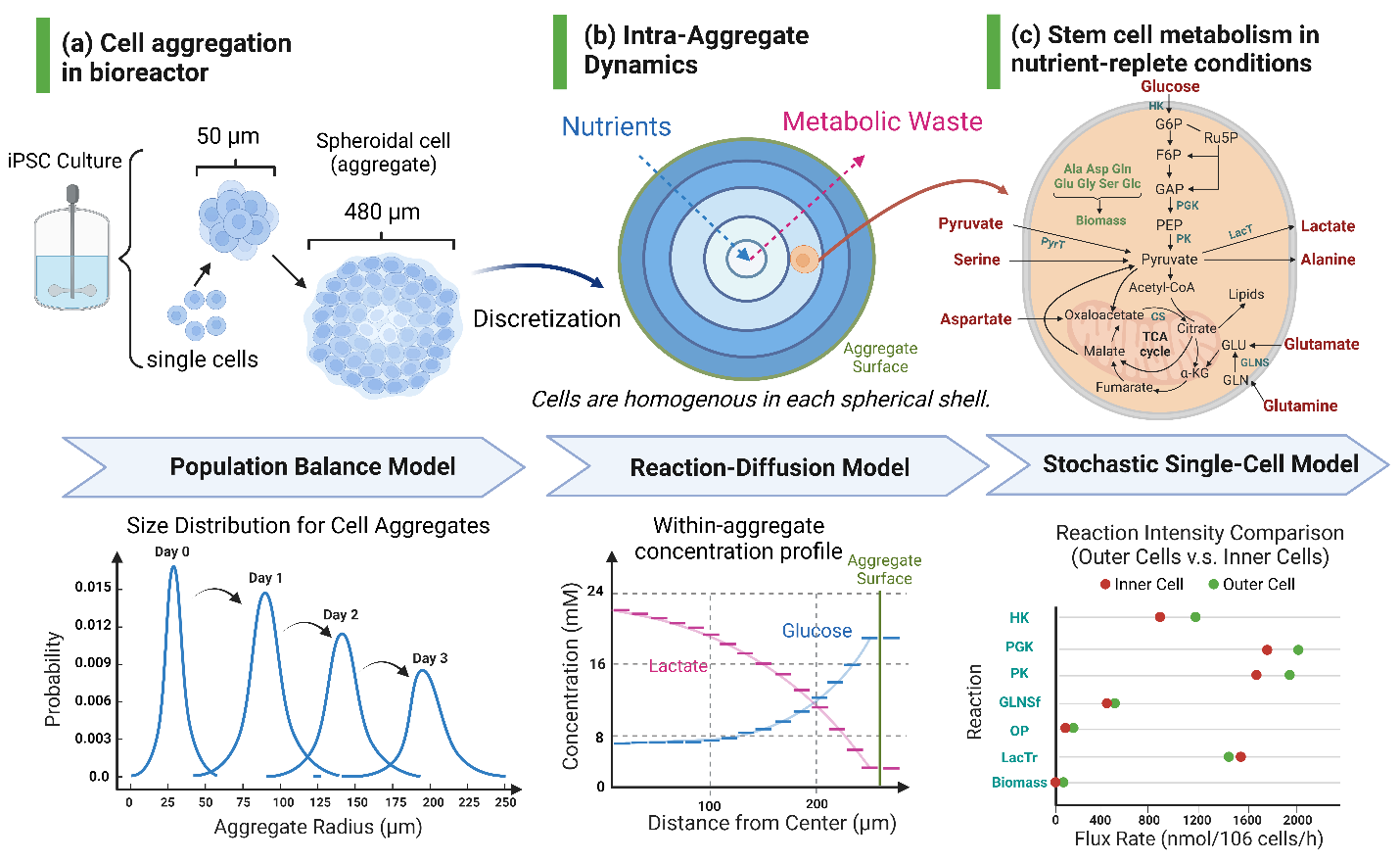
An illustration of our proposed biological system-of-systems (Bio-SoS) with modular design characterizing iPSC aggregate culture mechanisms, considering each cell as a complex system. (a) As the aggregates grow in size, spatial heterogeneity in microenvironment shaped by cell-cell interactions increases metabolic heterogeneity. (b) A reaction-diffusion model is used to characterize the intra-aggregate diffusion dynamics of nutrient and metabolite concentrations. Cell aggregates are spatially distributed into concentric shells. (c) The single-cell metabolic kinetic model describes cell response to microenvironmental variations. Metabolic heterogeneity is characterized by the difference in fluxes between exterior cells and inner cells locating at different positions in aggregates. In addition, a spatial variance analysis on the Bio-SoS is derived to quantify the impact of aggregate size on cell product quality heterogeneity.
Highlights of project NIIMBL 5.2T (ongoing):
-
Problem Statement
1) Large-scale production of induced pluripotent stem cells (iPSCs) is essential for cell therapies and regenerative medicines. Yet, iPSCs productivity and pluripotency are highly sensitive to culture conditions. Subtle changes in culture conditions can lead to stress and result in cell populations with heterogeneous differentiation potential.
2) The objectives of this project are to develop a robust online two-photon excitation (TPE) fluorescence optical sensor and knowledge graph and machine learning (KG-ML) algorithm that can enhance iPSC culture monitoring and control to improve critical quality attributes (CQAs), including pluripotency, growth rate, and productivity. -
Technology Development.
To achieve this goal and facilitate large-scale production of iPSCs, three tasks will be
conducted:
1) refine the two-photon excitation (TPE) fluorescence optical sensor to measure real-time intracellular redox state of iPSC cell-cultures, i.e., key enzymes NADH/NADPH/FAD for energetic metabolism;
2) gain knowledge of iPSC metabolism in static and bioreactor cultures with aggregates and on microcarriers; and
3) develop an improved KG-ML framework that can monitor and control iPSCs growth and pluripotency. The iPSC experiments will validate the performance of the TPE sensor and predictive power of the KG-ML. - Projected Impacts.
The value proposition of this project includes:
1) expediting the TPE sensor and interpretable KG-ML technology transfer to industry and workforce training programs;
2) accelerating intensified, reliable, automated, and scalable iPSC production in bioreactors; and
3) supporting Good Manufacturing Practice (GMP) development via QbD and automation.
NIIMBL 6.1G:Advanced FISH Assay and Mechanism Hybrid Surrogate to Improve mRNA Vaccine Potency Assessment and Prediction
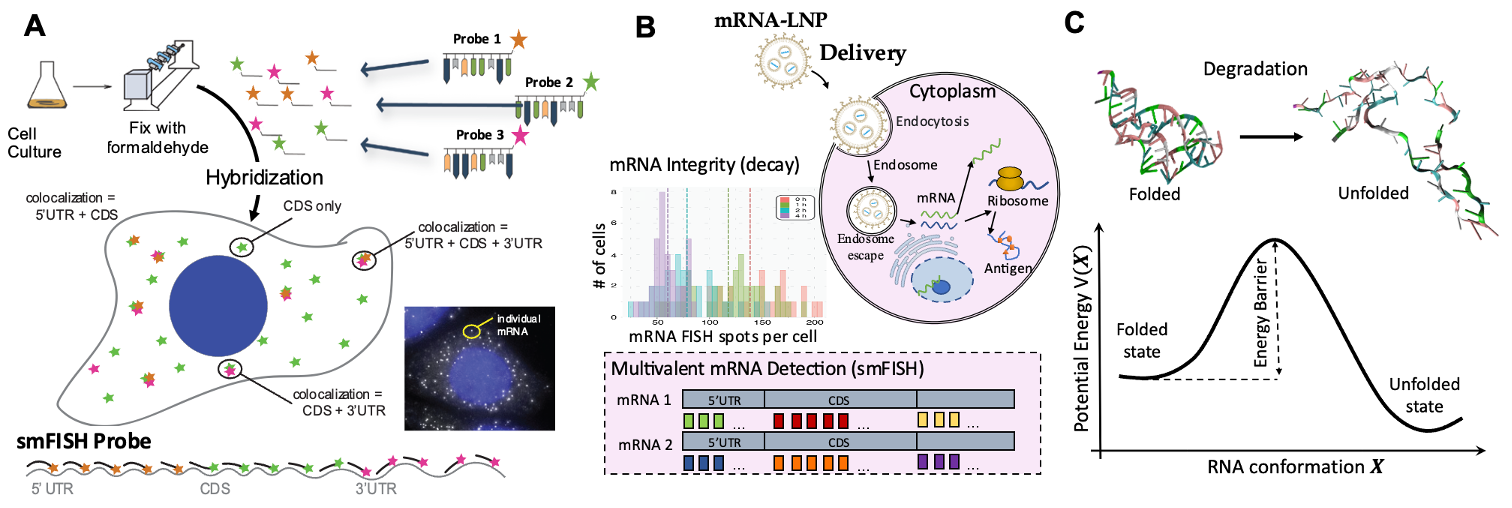
Coupled with the advancement of gene and protein expression assay technologies, we are developing a hybrid model and machine learning approaches to improve the prediction of mRNA vaccine potency and support gene therapy manufacturing quality control. (A) Schematic of smFISH protocol and colocalization. Three probes (located at 5¡¯UTR, CDS, 3¡¯UTR respectively) are prepared and conjugated to different fluorescent dyes so that it emits fluorescent light that can be observed through a microscope. (B) An illustration of monitoring RNA integrity, tracking critical transport pathways, and finding where RNA molecules go during multivalent mRNA delivery and translation process. (C) Development of a mechanistic hybrid model that can advance the scientific understanding of mRNA vaccine delivery, transfection, and translation process mechanisms, accounting for RNA folding and binding dynamics, and particle-particle interactions (e.g., RNA-RNA, RNA-protein, and RNA-ion).
Highlights of project NIIMBL 6.1G (ongoing):
-
Problem Statement
1) The COVID-19 pandemic has promoted rapid development of mRNA vaccines targeting a wide range of infectious diseases. The ongoing emergence of virus variants continuously challenges quality assurance/control (QC/QA) of mRNA vaccines. This enforces the critical need for a multiplexed potency assay and a mechanistic surrogate that can provide a reliable prediction on the potency and quickly screen multivalent mRNA vaccines encoding multiple proteins of variant strains.
2) The objective of this project is to develop and validate single-molecule RNA-fluorescence in situ hybridization (smFISH), mechanistic & hybrid surrogate model, and process analytical technologies (PATs) for mRNA delivery and translation processes to ensure vaccine efficacy. -
Technology Development. We focus on improving mRNA vaccine potency prediction during the delivery and
translation processes. The project includes three tasks:
1) conduct systematic experiments to validate the predictive power of smFISH, surrogate, and proposed PATs on mRNA vaccine potency, including tracking mRNA structure-function integrity, detecting RNA degradation, measuring the efficiency of mRNA delivery and antigen protein translation;
2) develop molecular dynamics and mechanistic models for mRNA stability and translation, incorporating RNA folding/binding, structure-function correlation, and particle-to-particle interactions (i.e., RNA-to-RNA, RNA-to-protein, RNA-to-lipid, RNA-to-ion);
3) develop a mechanistic and hybrid surrogate for the enzymatic biochemical reaction chain of integrated RNA delivery and translation processes.
- Projected Impacts.
The proposed smFISH assay, surrogate, and PATs can support QA/QC of a wide range of multivalent mRNA vaccines
and improve public health, including in low- and middle-income countries:
1) improve mRNA vaccine stability and delivery process consistency;
2) reduce waste during transportation and distribution;
3) form a quick response to new virus variants and ensure mRNA stability and efficacy;
4) accelerate the QA/QC screening for multivalent mRNA vaccine potency;
5) enhance the predictive power on the potency of new mRNA vaccines and the immune response of people with different genetic backgrounds.
Digital twin project for RNA manufacturing
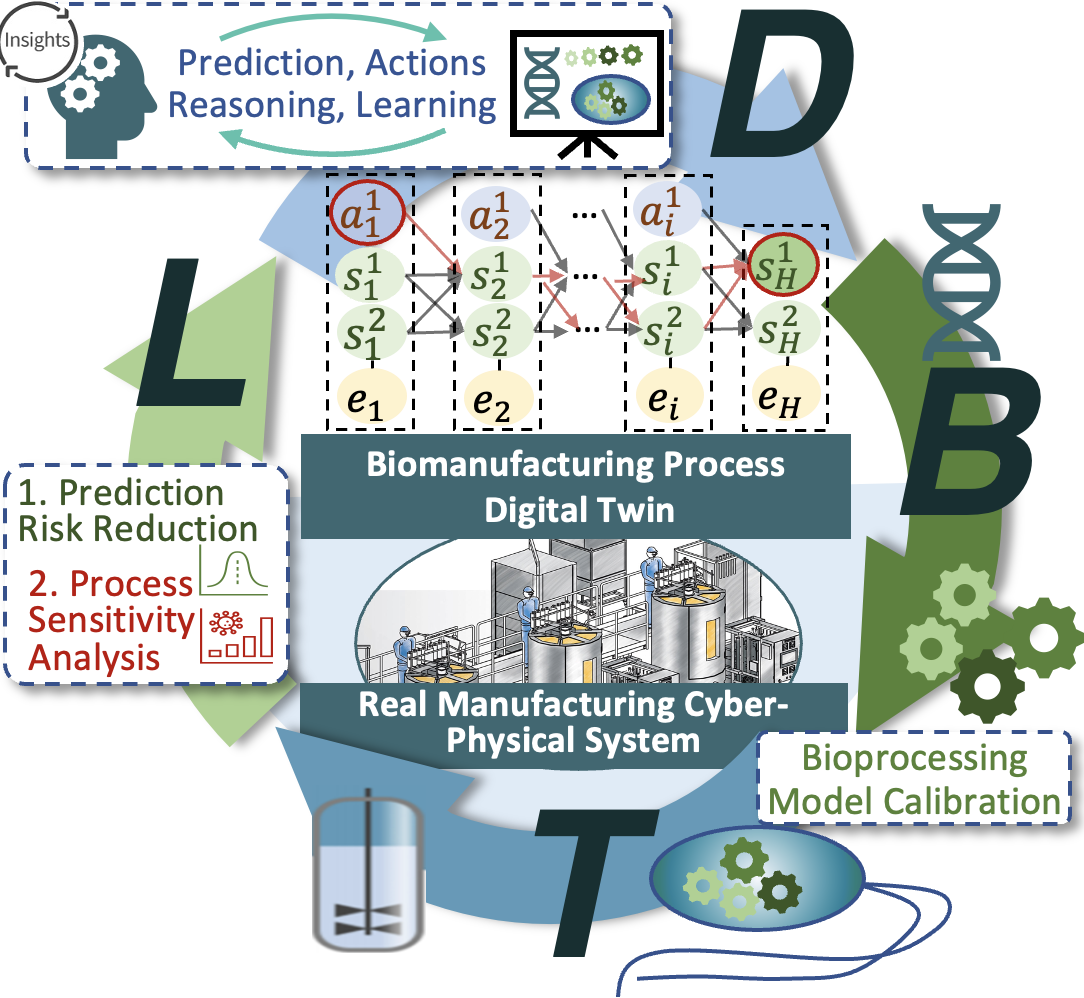
A multi-scale hybrid (¡°mechanistic + ML¡±) model and the digital twin characterize the underlying mechanisms of In Vitro (IVT) RNA synthesis process to improve the estimation of reaction rates and the prediction of production processes accounting for (A) molecule folding/binding, structure-function dynamics, and particle-to-particle interactions; and (B) enzymatic molecular reaction networks modeling the interdependent effect of multiple parameters and state inputs.
Highlights of the digital twin project (ongoing)
- Problem Statement. To support a rapid-response RNA vaccine manufacturing, we are providing an integrated digital platform enabling the fast optimal design of production processes and guiding the Design-Build-Test-Learn (DBTL) cycle for various RNA products
-
Technology Development. The project objective is to create an intelligent digital twin and process
analytical technologies (PATs) to provide risk- and science-based knowledge representation and accelerate
the development of flexible robust intensified automated RNA manufacturing processes.
1) Create a multi-scale bioprocess probabilistic knowledge graph (KG) hybrid (¡°mechanistic + ML¡±) model with modular design for end-to-end RNA synthesis process that can represent underlying mechanisms from an atomistic to molecular to macro-scale, facilitate data fusion, and guide an intelligent ¡°reuse¡± strategy to enhance rapid-response RNA vaccine manufacturing against new viral mutations.
2) Develop a Bayesian KG, accounting for model uncertainty, and risk-based predictive analytics that can guide sample efficient mechanism learning and support manufacturing process monitoring based on partially observed information.
3) Introduce optimal design of experiments to provide reliable reasoning as to where to sample next experiments, guide digital twin development, and support optimal process design/control to improve product quality consistency and yield. - Projected Impacts.
1) Facilitate the integration of heterogenous data and information collected from different RNA manufacturing processes for various RNA products.
2) Advance the scientific understanding of enzymatic RNA synthesis reaction mechanisms accounting for biomolecular structure-function dynamics.
3) Speed up the development of flexile, reliable, and automated RNA vaccine manufacturing processes for a rapid response to new viral outbreaks.